#technology
08th October, 2018
Machine Learning: Introduction and Techniques
Within traditional programming, input data is commonly processed using a collection of functions and linear instructions. It is the programmers objective to assign the correct logical paths and processes from which the machine can follow. These instructions are most often executed in sequential order, until the desired result is met. Applied refinement of modern software usually results in deceptively smart systems and applications. Though, the fundamentals of computing and software operation itself is inherently dumb. Due to software being mainly developed for specific tasks and operations, any ambiguous changes to input data outside of its programming will result in errors and/or exceptions. This issue becomes a substantial downfall to scripted programming, as it requires long term updates and support to exist in a dynamic environment. A direct answer to this issue is conceptualised within machine learning (ML). The architecture itself utilises the integration of augmented neural networks (ANN), which allows the machine to build an internal model and understanding of the data it collects. The program, or artificial intelligence (AI), can then build dynamic functions to cope within a dynamic environment; essentially granting the ability to learn. The need for auxiliary human programming then becomes redundant, as the machine becomes capable of assisting its own evolution and ongoing optimisation. Birth of a neuron Unlike traditional approaches to software development, every AI based on ANN will have to undergo development and training in order to become proficient in its task. Each new instance of machine learning begins with both input data and a ruling algorithm. The machine has to make sense of the input data, from which it constructs neurons and pathways in a bid to progress. The end goal algorithm is used as a set of instructions, so the AI can decide on what task each neuron will perform. The result of this, is an interconnected web of neurons, which handles the processing of data. This web of neurons can then be used as a dynamic interface that aims to achieve the end goal. An example of this can be translated through training a machine to play the classic Nintendo video game, Super Mario. Due to the in-game progression being mainly linear in its side scrolling nature, it becomes a suitable candidate for exampling a neural network. For an overall set goal, the AI would need only understand that the amount of distance travelled east directly correlates with the overall progression. Only slight additional parameters can then be introduced to refine the end goal, such as time taken, and amount of deaths experienced. These parameters can be useful, as they are used to gauge the overall development, or ‘fitness level’ of the AI. Due to the dynamic development of neural networks, each new instance of ML will also most likely produce independent and contrasting results. To improve the efficiency of global development, multiple simultaneous instances of the same AI can be ran in order to increase the chance of developing higher performing sets of neurons. Some instances will learn faster than others: and others will approach the task in different ways. This also allows for insightful and interesting results between developing instances… The initial application and development of this process is referred to as the first generation. Within this generation of grouped instances, the in-game character would mostly struggle to perform any significant progress - mostly standing still, or making sporadic movements. This is the AI trying to propagate neurons and map the available controls to logical functions. One neuron, for example, may be used to move the character further right, whilst another makes the character jump. The more complex a network becomes, the more likely it will be able to succeed in the overall task. Further complexity is then left to develop until a progressional bottleneck is reached. β¨It is at this point where the performance of each instance is analysed and compared, allowing the most desired set of neurons to be selected for merging. The succeeding candidates will have their neural networks merged, allowing specific strengths to be unified into one entity. Not only does this help in excluding any unwanted behaviour and developments, but also acts as a form of natural selection. The task of merging is usually performed by humans in low-end and developing instances of machine learning. However, additional AIs can be assigned to this task, allowing the process to become completely automated. The merging of these networks is also integrated with some small mutations, which is very similar to natural evolution. This allows for some experimental deviations and guaranteed changes between ongoing evolutions. This first evolution, or second generation, will begin learning with all the best neural paths inherited from their parents. It is this merging of neurons which most always breaks through bottlenecks and allows another generation to excel past the successes of their parents. This is a direct example of the machine becoming more intelligent. Unlike natural evolution, where only genes are passed to subsequent generations, machine learning directly allows the next generation to extend and build upon fully developed neural networks… The technical term for this process is referred to as neuro evolution. In our example of Super Mario, subsequent generations will increasingly progress through the level at ease. Once the machine is able to complete a level without deaths, the AI could be left to either optimise its current processes, or be reassigned additional algorithms, from which it can further progress. As the machine is able to quickly master such simple games, the point of advanced execution is reached somewhat exponentially. AIs then have the ability to far exceed the skill and efficiency of humans, which make them a very desirable technology to automate tasks. “Our intuition about the future is linear. But the reality of information technology is exponential, and that makes a profound difference. If I take 30 steps linearly, I get to 30. If I take 30 steps exponentially, I get to a billion.” - Ray Kurzweil Further Learning Another method used in training ML based AI is via reinforcement learning. As the term suggests, the AI builds up an understanding of the input data through boolean (true or false) feedback. This is achieved by returning the final output back into the AI, where the data is stored within the internal model for future reference and comparison. Over time, the machine can then become more efficient at handling dynamic inputs, as the internal modal continues to build a wider understanding of the data. To present a digestible example, an image identifier application can be used in showcasing the logic involved. For this in particular, the machine will only be asked to identify pictures of either a single cat or dog. Using two photos of the respective animals in the base model, the machine can operate with less need for advanced algorithms, as it can use this data for an initial learning reference. Within the dynamic learning process, additional images of both cats and dogs are then ran through the input stream. The machine can immediately start producing probabilities based on the initial images, which are then assessed and returned to the machine as reinforcement learning. However, even with minimal feedback, the machine can reach relatively advanced levels of identification through its neural network alone. The instance can develop some unique neurons, which identify certain features of the animals, as subtle as they may be. This, combined with an internal model and ongoing feedback, results in quite a powerful methods of implementing machine learning. “By far, the greatest danger of Artificial Intelligence is that people conclude too early that they understand it.” - Eliezer Yudkowsky Outcomes The human brain is estimated to have on average 100 billion neurons, with each neuron connected to around another 1000 neurons. In our examples of a simple neural networks, there will only be a handful of neurons that directly deal with the input data itself. For a network as complex as the human brain to be simulated, it would require a computer at least 3.5 times more powerful than the most powerful computer on earth. As interesting and impressive as machine learning can be, it is still considered ‘weak AI’ in comparison to other developing AI technologies. The concept of ML is regarded as the next level of automation, rather than significant intelligence. However, the potentials of ML are still staggering and can easily surpass human abilities within specific tasks. The technology must be monitored and respected, as abuse of such things can result in repercussions that arise at exponential rates. Due to Moors law and the advent of affordable and powerful processors, ML is increasingly used within a plethora of industries. The trend in later years has seen companies in many governing and commercial sectors adopt ML into their core technologies. This trend will more than likely continue in years to come, as the technology has great potential. “The pace of progress in artificial intelligence (I’m not referring to narrow AI) is incredibly fast. Unless you have direct exposure to groups like Deepmind, you have no idea how fast—it is growing at a pace close to exponential. The risk of something seriously dangerous happening is in the five-year timeframe. 10 years at most.” - Elon Musk
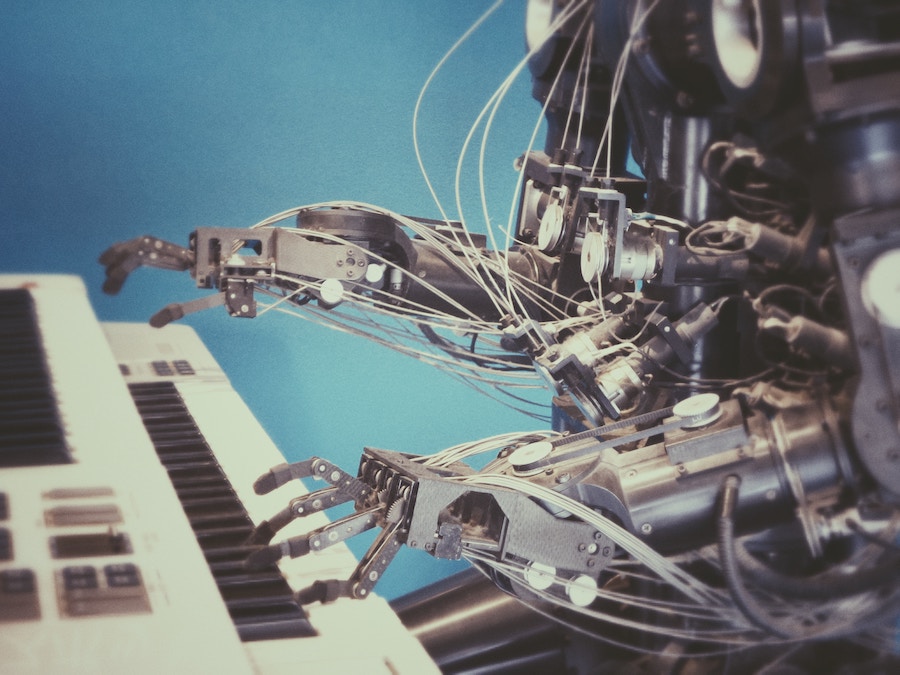